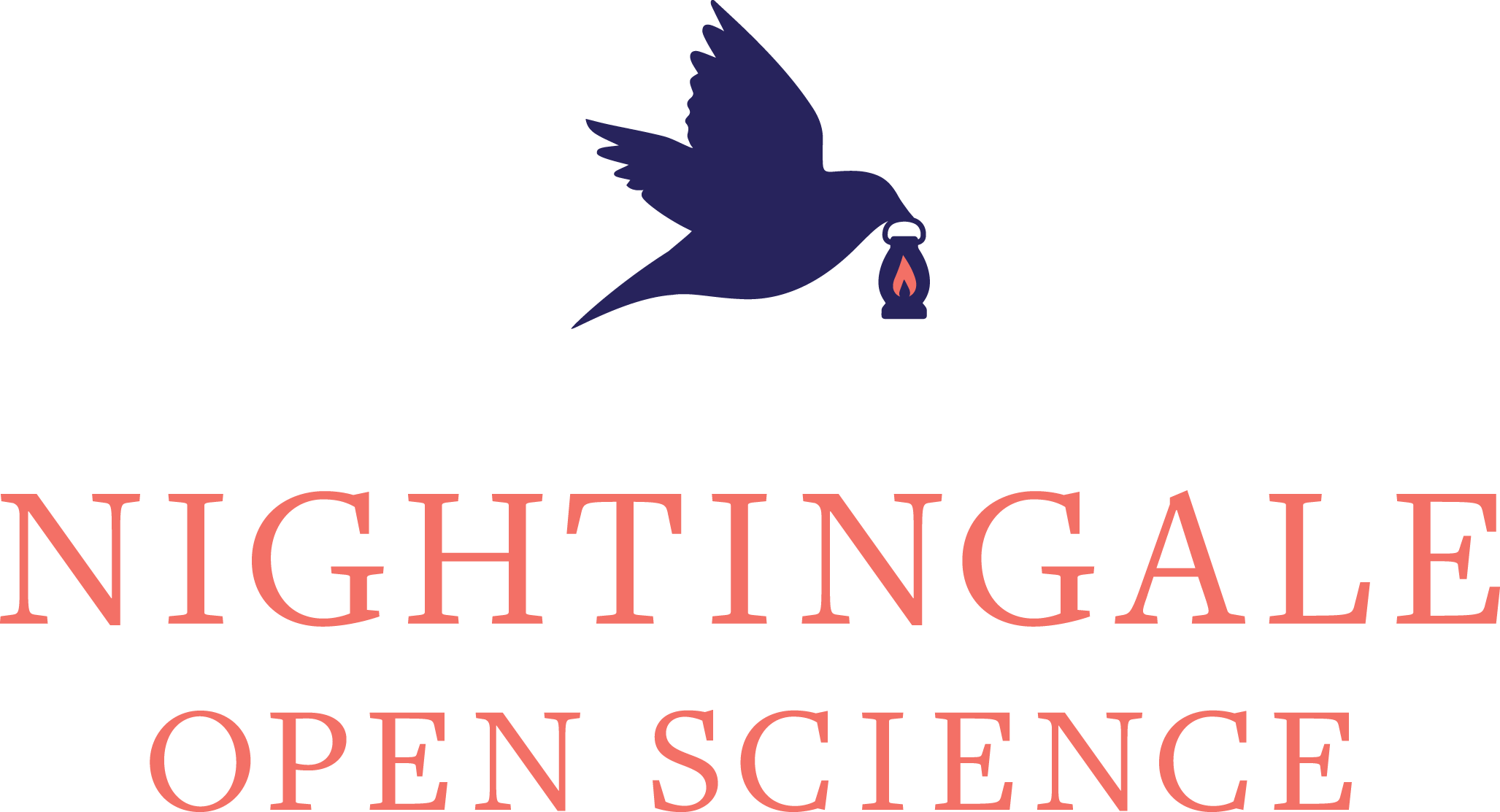
Learn more about Nightingale DATA
Thank you! Your submission has been received!
Oops! Something went wrong while submitting the form.
More than 10 million people fall ill with active tuberculosis (TB) each year and more than 1.5 million die due to the disease every year despite it being a preventable, treatable, and curable disease. It is among the oldest diseases observed in prehistoric human remains from as far back as 4,000 BCE and animal remains from 17,000 BCE. It was among the leading causes of death even a century ago. Despite the creation of the BCG vaccine 100 years ago, it is the leading infectious cause of death today, excluding the COVID-19 pandemic. It is the leading cause of death in those with HIV disease and a major cause of deaths due to antimicrobial resistance.
The UN Sustainable Development Goals seeks to achieve a 50% reduction in the TB incidence rate and a 75% reduction in deaths due to TB from 2015 to 2025. Despite achieving a 2% reduction in TB infection every year for the last two decades, these efforts have been upended by the COVID-19 pandemic. The number of newly diagnosed cases has fallen from 7.1 million in 2019 to 5.8 million in 2020 due to disruptions in health services caused by the COVID-19 pandemic.[1] These numbers have partially recovered to 6.4 million in 2021, but an estimated 4 million new patients with active tuberculosis disease remain undiagnosed. This drop has reversed WHO’s STOP TB efforts back to 2012 levels. Undiagnosed and untreated cases further the spread of the disease, and the disruption caused by the pandemic is feared to increase the number of new TB infections in the next few years.
Microscopic examination of the sputum smear for acid-fast bacilli is a common test for the diagnosis of tuberculosis, especially in resource-constrained areas. Despite the low sensitivity of about 60% to diagnose pulmonary tuberculosis, it is a cheap and effective diagnostic technique that can be deployed at scale to control tuberculosis infections and their spread. However, there is limited availability of trained microbiologists to interpret the slide images at scale in various parts of the world. Digitized microscopy combined with artificial intelligence algorithms is proposed as a solution to this problem, and it can be deployed at scale in resource-constrained settings where frontline health workers can be trained to collect the specimen and prepare the slides.
The first commercial system (by Wellgen Medical) in a peer-reviewed journal was by Huang et al. in 2022, who reported that the automation system achieved significantly higher TB smear sensitivity and laboratory efficiency.[4] Results showed that, compared to manual microscopy, the automation system’s performance of accuracy, sensitivity, and specificity was 95.7% (1,651/1,726), 87.7% (57/65), and 96.0% (1,594/1,661), respectively. The negative predictive value (PPV) was 97.8% at a prevalence of 8.2%.
WellGen Medical graciously agreed to make the TB microscopy images available as an open data set through the Nightingale Platform with generous support from the Gordon and Betty Moore Foundation’s Diagnostic Excellence Initiative. We believe that this data set will spur novel AI algorithms for the detection of tuberculosis, which may then be deployed at scale to diagnose and treat tuberculosis. We also believe that such innovations will lead to AI-based algorithms for other microorganisms as well.
The data dictionary for the Detecting Active Tuberculosis data set is published here, and the data set is available on the platform to registered users. The data set has more than 105,000 microscopy images of which about 5% are positive for tuberculosis.[4] Nightingale users may immediately start accessing this data set to build computer vision algorithms to detect tuberculosis bacilli in microscopy slides.
In addition to ML scientists, we invite clinicians to our platform to engage in collaborative research by providing clinical guidance, validating the ML models, or for labeling the training and validation data. Our discussion forums serve as a medium for collaboration between our community of users.
A future version of this data set will include more than 1 million images. We also plan to host a machine learning challenge for the creation of novel ML algorithms to detect the tubercle bacillus. Furthermore, Nightingale is working on deploying a data labeling system on our platform based on user feedback. Join the Nightingale platform to learn and conduct medical ML research. Subscribe to our mailing list at the bottom of this page to stay informed.
References
1 World health statistics 2023: monitoring health for the SDGs, sustainable development goals. Geneva: World Health Organization; 2023.
2 Global report on neglected tropical diseases 2023. Geneva: World Health Organization; 2023.
3 Lin YE, Foster N, Juergens N, Nachimuthu S, Risley J, Haynes K, et al. Detecting Active Tuberculosis Bacilli on TB Smears [Internet]. 2023.
4 Huang H, Kuo K, Lo M, Chou H, Lin YE. Novel TB smear microscopy automation system in detecting acid-fast bacilli for tuberculosis - A multi-center double blind study. Tuberculosis. 2022 18 May;135:102212.