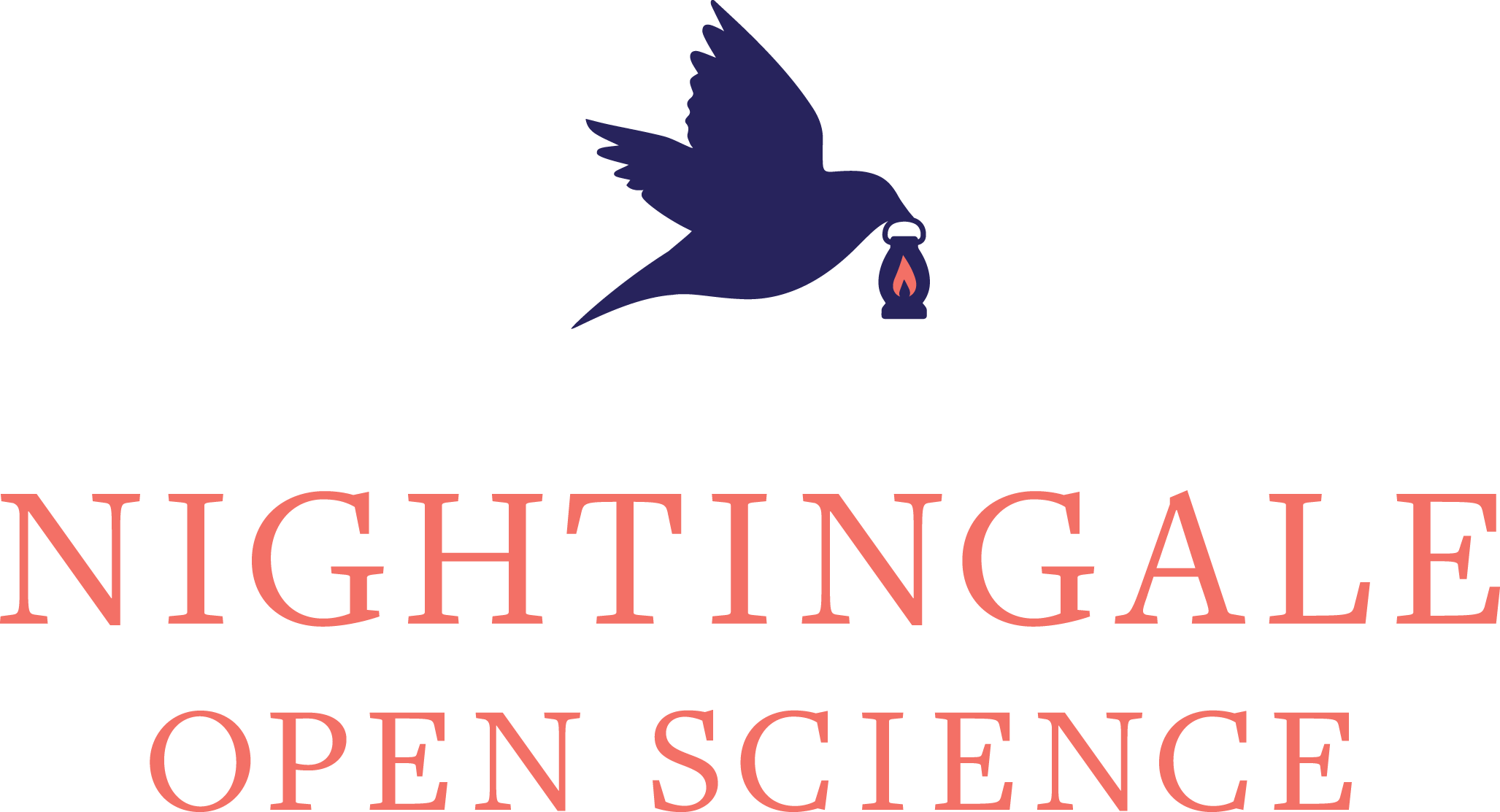
Learn more about Nightingale DATA
Thank you! Your submission has been received!
Oops! Something went wrong while submitting the form.
Every year, 40 million women get a mammogram; some go on to have an invasive biopsy to better examine a concerning area. Underneath these routine tests lies a deep—and disturbing—mystery. Since the 1990s, we have found far more ‘cancers’, which has in turn prompted vastly more surgical procedures and chemotherapy. But death rates from metastatic breast cancer have hardly changed.
When a pathologist looks at a biopsy slide, she is looking for known signs of cancer: tubules, cells with atypical looking nuclei, evidence of rapid cell division. These features, first identified in 1928, still underlie critical decisions today: which women must receive urgent treatment with surgery and chemotherapy? And which can be prescribed “watchful waiting”, sparing them invasive procedures for cancers that would not harm them? There is already evidence that algorithms can predict which cancers will metastasize and harm patients on the basis of the biopsy image. Fascinatingly, these algorithms also hone in on features that humans neglect, for example, the nature of the non-cancerous tissue surrounding the tumor. But to date, the datasets linking biopsy images to patient outcomes—metastasis, death—have been far smaller than what is needed to apply modern approaches.
Breast cancer health disparities and inequities exist on many levels, including race, ethnicity, socioeconomic status, geography, and access to healthcare. For example, Black women are more likely to die from breast cancer than white women, despite having a lower incidence of the disease. This is due to a combination of factors, including differences in access to healthcare, late diagnosis, and more aggressive forms of the disease.Triple negative breast cancers (TNBC) are characterized by the absence or low levels of estrogen receptors (ER), progesterone receptors (PR), and human epidermal growth factor 2 receptors (HER2) on the tumor cells. According to the American Cancer Society, tThey constitute about 15% to 20% of breast cancers and are among the hardest subtype to treat. They have higher incidence in African American and Hispanic women, with African Americans facing worse outcomes than other race and ethnic groups.
NIH AIM-AHEAD and Nightingale developed this challenge in order to catalyze the development of algorithms that find new signals in digital pathology images, ultimately providing new insights into which minority patients may be at risk and need preventive treatment.
The goal of this challenge is to predict the stage of minority patient’s cancers, using only the slide images generated by a breast biopsy.
Cash prizes of $5,000 will be awarded to winners, and they will be invited to attend the AIM-AHEAD conference in Bethesda, MD in August 2023. AIM-AHEAD will support travel to the invited teams. In accordance with NIH AIM-AHEAD policy, only US citizens and permanent residents are eligible to participate in this challenge and be eligible for prizes. We strongly suggest that each team include some members from communities underrepresented in data science and artificial intelligence and machine learning.
How to participate?
1. Users meeting AIM-AHEAD criteria must first register for the challenge through AIM-AHEAD Connect (link below)
2. After registering with AIM-AHEAD, you will receive an email with a link to the Nightingale contest page to join the contest. The contest page describes the rules, prizes, and how to request free compute credits.
3. You must be a registered Nightingale user to join the contest. It takes 1 business day to admit academic users to our platform.
4. You may invite other AIM-AHEAD and Nightingale registrants to join your team. All team members have access to your team project.
Tutorials
Those who are new to machine learning are encouraged to watch and do the hands-on tutorials for a quick introduction to machine learning. The tutorial also gives you an introduction to the Nightingale Platform.
Visit the AIM-AHEAD website to join this challenge. Registration ends May 31. Challenge ends June 23.
https://www.aim-ahead.net/data-science-training-core/2023-health-equity-data-challenge/